
Web Application Design
Web Application Design
Empowering Insightful Decision Making
Empowering Insightful Decision Making
Empowering Insightful Decision Making
Hatari's ML Ops was designed to enchance insights for data transparency and Model Optimization.
Hatari's ML Ops was designed to enchance insights for data transparency and Model Optimization.
Hatari's ML Ops was designed to enchance insights for data transparency and Model Optimization.
Summary
Summary
01
Role
Product designer, product, product strategist and interaction designer
02
Overview
We conducted user interviews, surveys, and analyzed in-app analytics to understand the pain points and user needs. We also studied competitor apps and industry trends to gather insights
03
Challenge
We conducted user interviews, surveys, and analyzed in-app analytics to understand the pain points and user needs. We also studied competitor apps and industry trends to gather insights
04
What I did
We conducted user interviews, surveys, and analyzed in-app analytics to understand the pain points and user needs. We also studied competitor apps and industry trends to gather insights
05
Takeaway
We conducted user interviews, surveys, and analyzed in-app analytics to understand the pain points and user needs. We also studied competitor apps and industry trends to gather insights

01
Role
Product designer, product, product strategist and interaction designer
02
Overview
We conducted user interviews, surveys, and analyzed in-app analytics to understand the pain points and user needs. We also studied competitor apps and industry trends to gather insights
03
Challenge
We conducted user interviews, surveys, and analyzed in-app analytics to understand the pain points and user needs. We also studied competitor apps and industry trends to gather insights
04
What I did
We conducted user interviews, surveys, and analyzed in-app analytics to understand the pain points and user needs. We also studied competitor apps and industry trends to gather insights
05
Takeaway
We conducted user interviews, surveys, and analyzed in-app analytics to understand the pain points and user needs. We also studied competitor apps and industry trends to gather insights

01
Role
Product designer, product, product strategist and interaction designer
02
Overview
We conducted user interviews, surveys, and analyzed in-app analytics to understand the pain points and user needs. We also studied competitor apps and industry trends to gather insights
03
Challenge
We conducted user interviews, surveys, and analyzed in-app analytics to understand the pain points and user needs. We also studied competitor apps and industry trends to gather insights
04
What I did
We conducted user interviews, surveys, and analyzed in-app analytics to understand the pain points and user needs. We also studied competitor apps and industry trends to gather insights
05
Takeaway
We conducted user interviews, surveys, and analyzed in-app analytics to understand the pain points and user needs. We also studied competitor apps and industry trends to gather insights

01
Role
Product designer, product, product strategist and interaction designer
02
Overview
We conducted user interviews, surveys, and analyzed in-app analytics to understand the pain points and user needs. We also studied competitor apps and industry trends to gather insights
03
Challenge
We conducted user interviews, surveys, and analyzed in-app analytics to understand the pain points and user needs. We also studied competitor apps and industry trends to gather insights
04
What I did
We conducted user interviews, surveys, and analyzed in-app analytics to understand the pain points and user needs. We also studied competitor apps and industry trends to gather insights
05
Takeaway
We conducted user interviews, surveys, and analyzed in-app analytics to understand the pain points and user needs. We also studied competitor apps and industry trends to gather insights

The Design Process
The Design Process
Introduction
Introduction
The why
The why
The why
Take your love of food to the next level.
The ML Ops feature was added to Hatari to help users better handle and enhance their machine learning models. With this feature, users can see how transactions are scored, keep track of their models' performance, and quickly adjust them as necessary. It's like giving users a toolbox to fine-tune their models and stay ahead of the game. This way, users can make smarter decisions and adapt to any changes in Hatari with confidence.
The ML Ops feature was added to Hatari to help users better handle and enhance their machine learning models. With this feature, users can see how transactions are scored, keep track of their models' performance, and quickly adjust them as necessary. It's like giving users a toolbox to fine-tune their models and stay ahead of the game. This way, users can make smarter decisions and adapt to any changes in Hatari with confidence.
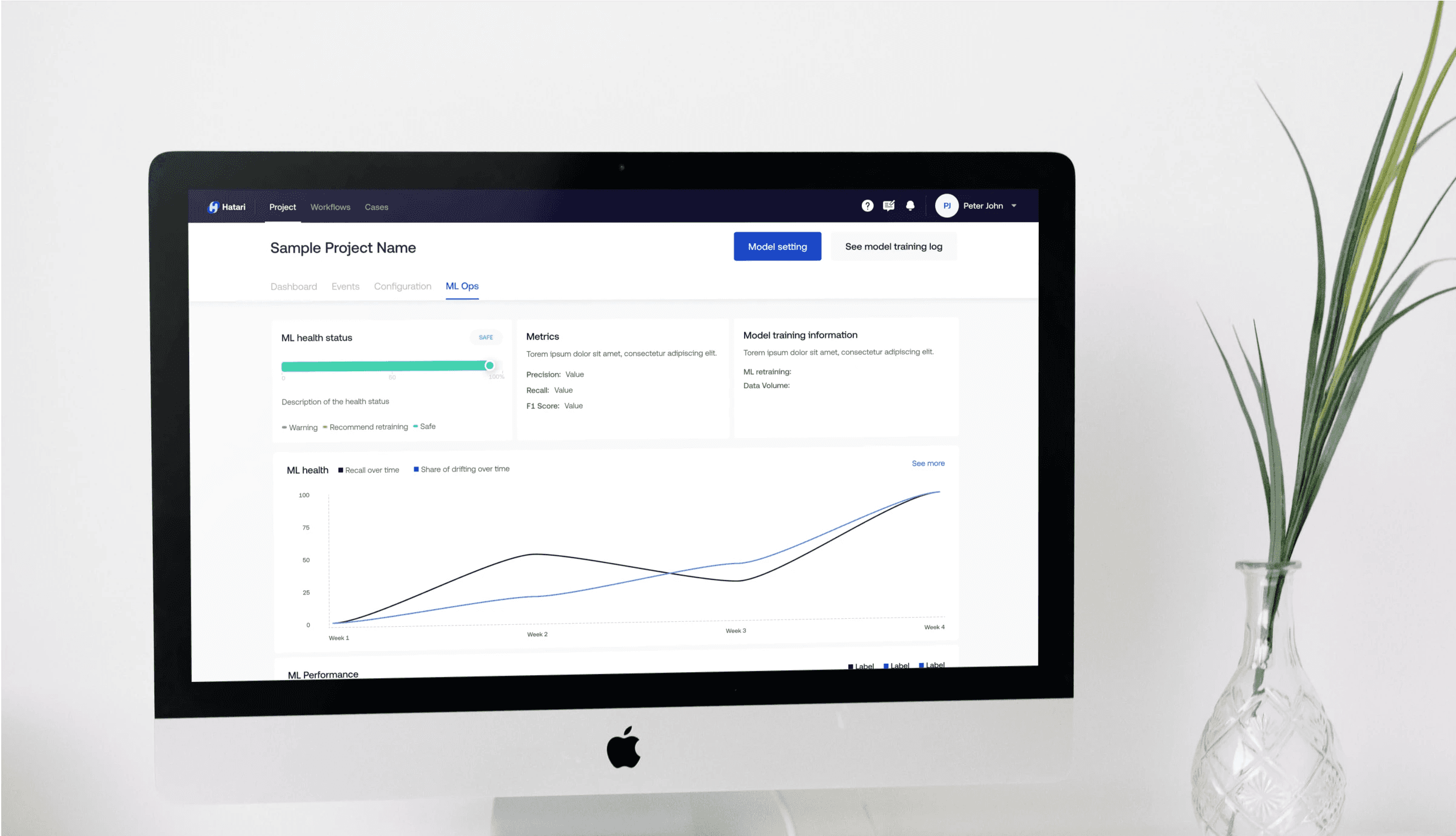
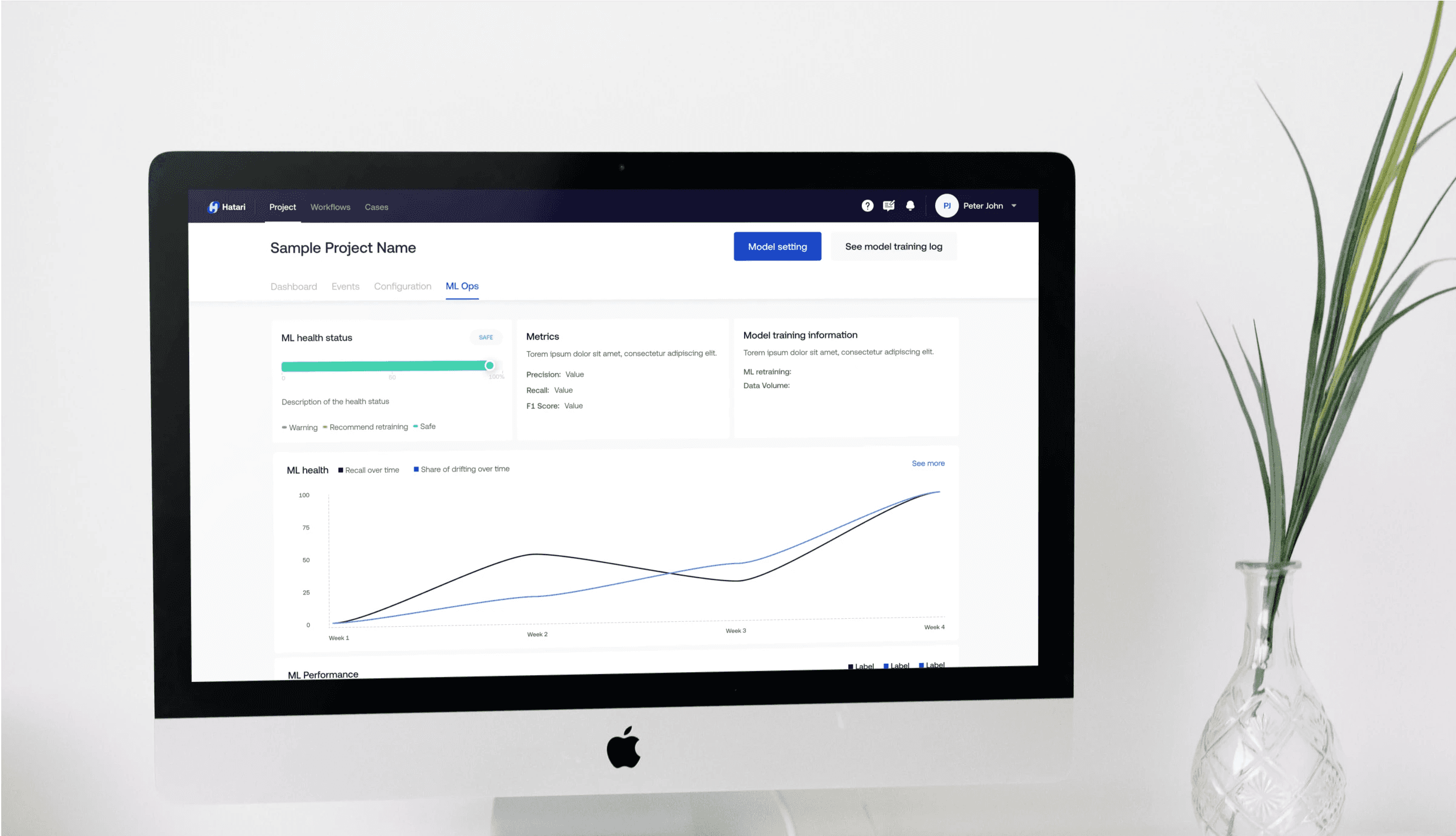
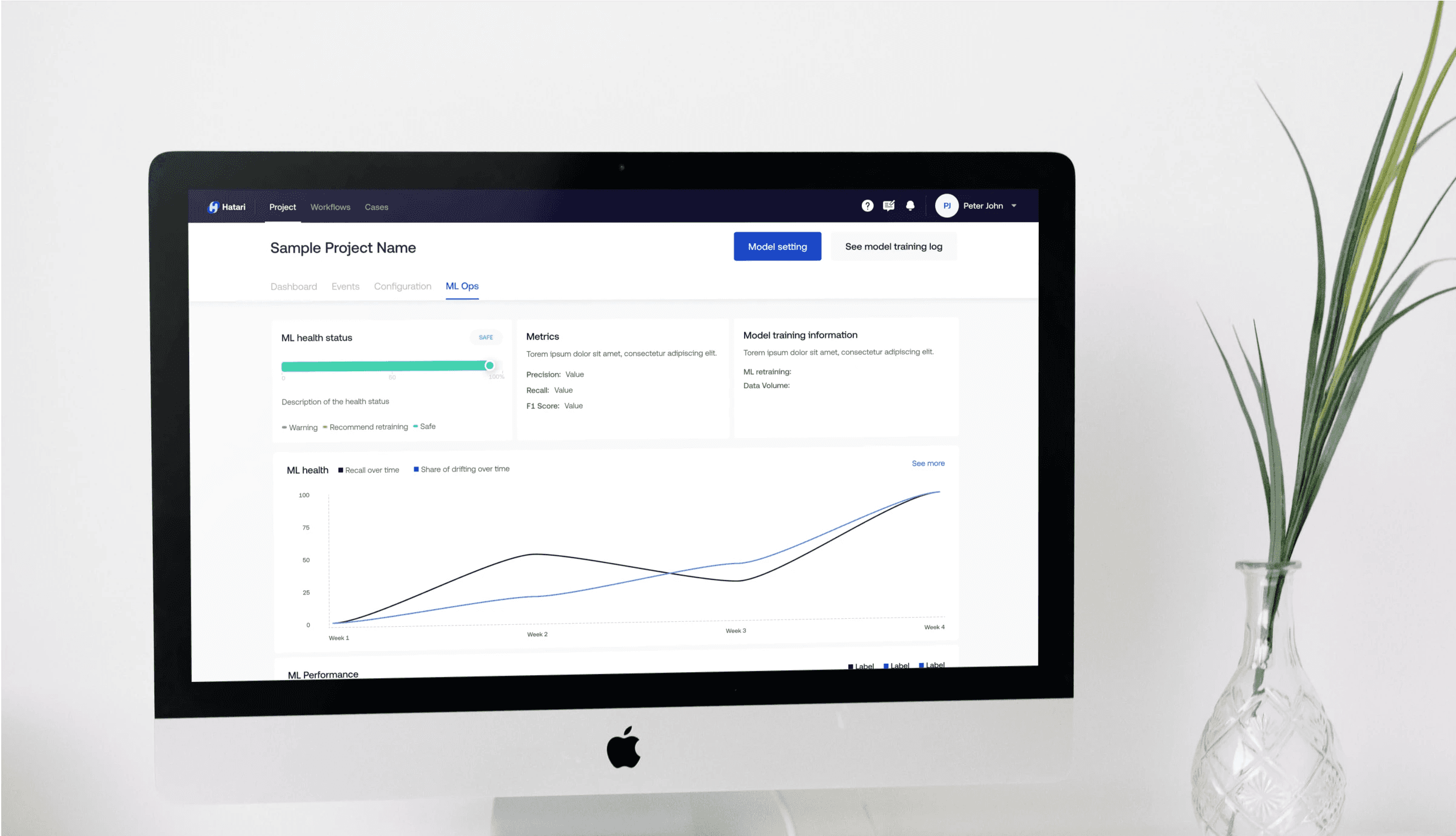
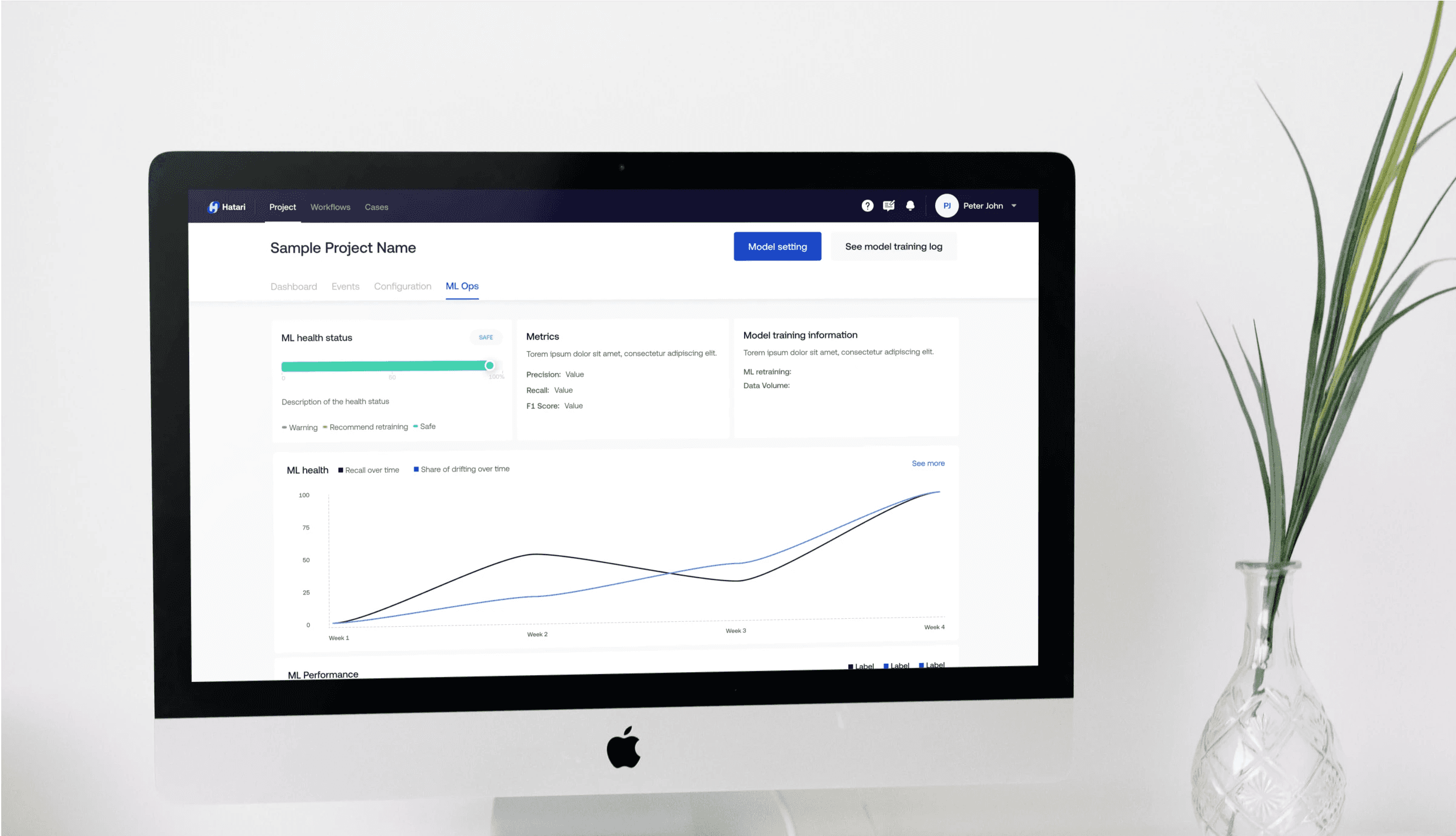
Target audience
Target audience
Understanding and identifying the users
Understanding and identifying the users
Understanding and identifying the users
Understanding and identifying the users
To create a useful ML Ops feature for Hatari, we needed to know who would use it. We talked to different kinds of users, like data scientists and developers, to understand what they needed. By learning about their work and what they struggled with, we made sure the feature would be helpful for everyone who uses Hatari to manage machine learning models.
To create a useful ML Ops feature for Hatari, we needed to know who would use it. We talked to different kinds of users, like data scientists and developers, to understand what they needed. By learning about their work and what they struggled with, we made sure the feature would be helpful for everyone who uses Hatari to manage machine learning models.
User research
User research
Talking to potential users
Talking to potential users
Talking to potential users
Talking to potential users
Before diving into designing the ML Ops feature for Hatari, it was important to talk directly to potential users. We reached out to different people who might use this feature, like data scientists and developers. By having conversations with them, we learned about their experiences, challenges, and what they wanted from a tool like Hatari. These discussions helped us gain valuable insights into their workflows, allowing us to design a feature that would truly meet their needs and make their jobs easier.
Before diving into designing the ML Ops feature for Hatari, it was important to talk directly to potential users. We reached out to different people who might use this feature, like data scientists and developers. By having conversations with them, we learned about their experiences, challenges, and what they wanted from a tool like Hatari. These discussions helped us gain valuable insights into their workflows, allowing us to design a feature that would truly meet their needs and make their jobs easier.
In the comprehensive user research step several valuable insights could be gathered. Here's some of what people had to saw:
In the comprehensive user research step several valuable insights could be gathered. Here's some of what people had to saw:
In the comprehensive user research step several valuable insights could be gathered. Here's some of what people had to saw:
In the comprehensive user research step several valuable insights could be gathered. Here's some of what people had to saw:
"Current tools lack integration capabilities with our existing infrastructure, causing data silos and inefficiencies."
"Current tools lack integration capabilities with our existing infrastructure, causing data silos and inefficiencies."
"Current tools lack integration capabilities with our existing infrastructure, causing data silos and inefficiencies."
"Current tools lack integration capabilities with our existing infrastructure, causing data silos and inefficiencies."
"Improving visualization tools could enhance our ability to interpret model outputs and make informed decisions."
"Improving visualization tools could enhance our ability to interpret model outputs and make informed decisions."
"Improving visualization tools could enhance our ability to interpret model outputs and make informed decisions."
"Improving visualization tools could enhance our ability to interpret model outputs and make informed decisions."
"Having a centralized dashboard for model monitoring and a streamlined process for model deployment are crucial for our workflow."
"Having a centralized dashboard for model monitoring and a streamlined process for model deployment are crucial for our workflow."
"Having a centralized dashboard for model monitoring and a streamlined process for model deployment are crucial for our workflow."
"Having a centralized dashboard for model monitoring and a streamlined process for model deployment are crucial for our workflow."
"A more efficient ML Ops solution could significantly reduce our time spent on manual tasks, allowing us to focus on model refinement and innovation."
"A more efficient ML Ops solution could significantly reduce our time spent on manual tasks, allowing us to focus on model refinement and innovation."
"A more efficient ML Ops solution could significantly reduce our time spent on manual tasks, allowing us to focus on model refinement and innovation."
"A more efficient ML Ops solution could significantly reduce our time spent on manual tasks, allowing us to focus on model refinement and innovation."
Brainstorming
Brainstorming
Bouncing ideas off each other
Bouncing ideas off each other
Bouncing ideas off each other
Bouncing ideas off each other
During brainstorming for the ML Ops feature in Hatari, our team collaborated to explore ideas such as improving model visualization tools and automating model retraining. We also discussed potential integrations with existing tools to streamline workflows. Through this process, we generated innovative concepts that laid the groundwork for further development.
During brainstorming for the ML Ops feature in Hatari, our team collaborated to explore ideas such as improving model visualization tools and automating model retraining. We also discussed potential integrations with existing tools to streamline workflows. Through this process, we generated innovative concepts that laid the groundwork for further development.




High Fidelity Design
High Fidelity Design
ML Ops for Hatari
ML Ops for Hatari
ML Ops for Hatari
ML Ops for Hatari
Our high fidelity prototype in Hatari is geared towards seamlessly integrating ML Ops functionalities into the platform. It begins with a user-friendly login process, followed by project creation and configuration, where users can upload data, connect streams, and train ML models.
Our high fidelity prototype in Hatari is geared towards seamlessly integrating ML Ops functionalities into the platform. It begins with a user-friendly login process, followed by project creation and configuration, where users can upload data, connect streams, and train ML models.
At the core of the design is the ML Panel tab, providing users with real-time insights into their ML model health. This ensures users can monitor model health effectively, with visual indicators prompting action if anomalies arise.
At the core of the design is the ML Panel tab, providing users with real-time insights into their ML model health. This ensures users can monitor model health effectively, with visual indicators prompting action if anomalies arise.
We delved into the realm of model retraining, offering users flexible options to configure retraining settings based on predefined drift levels. Users can opt for automatic retraining, where Hatari saves a fixed volume of data and triggers retraining upon detecting drift. Alternatively, users retain control with manual retraining, enabling them to define parameters such as data volume required, drift level threshold, and access a comprehensive log of model retraining activities.
We delved into the realm of model retraining, offering users flexible options to configure retraining settings based on predefined drift levels. Users can opt for automatic retraining, where Hatari saves a fixed volume of data and triggers retraining upon detecting drift. Alternatively, users retain control with manual retraining, enabling them to define parameters such as data volume required, drift level threshold, and access a comprehensive log of model retraining activities.
Takeaways
Takeaways
What I learnt from this
What I learnt from this
What I learnt from this
What I learnt from this
My takeaway from designing Hatari's ML OPS feature was the importance of user-centricity and collaboration in creating impactful solutions. By understanding user needs and working closely with diverse teams, I learned how to translate complex technical concepts into intuitive designs that enhance user experience and efficiency. This experience reinforced the value of iterative design processes and the significance of maintaining brand identity throughout product development. Overall, it highlighted the power of teamwork and empathy in driving successful product design.
My takeaway from designing Hatari's ML OPS feature was the importance of user-centricity and collaboration in creating impactful solutions. By understanding user needs and working closely with diverse teams, I learned how to translate complex technical concepts into intuitive designs that enhance user experience and efficiency. This experience reinforced the value of iterative design processes and the significance of maintaining brand identity throughout product development. Overall, it highlighted the power of teamwork and empathy in driving successful product design.